Expo
view channel
view channel
view channel
view channel
view channel
view channel
view channel
view channel
view channel
Clinical Chem.
HematologyImmunologyMicrobiologyPathologyTechnologyIndustry
Events
- Automated Decentralized cfDNA NGS Assay Identifies Alterations in Advanced Solid Tumors
- Mass Spectrometry Detects Bacteria Without Time-Consuming Isolation and Multiplication
- First Comprehensive Syphilis Test to Definitively Diagnose Active Infection In 10 Minutes
- Mass Spectrometry-Based Monitoring Technique to Predict and Identify Early Myeloma Relapse
- ‘Brilliantly Luminous’ Nanoscale Chemical Tool to Improve Disease Detection
- AI-Powered Platform Enables Rapid Point-of-Care Cancer Testing
- Calprotectin Testing Predicts Future Atherosclerotic Heart Disease Risk
- Low-Cost Blood Test Enables Early Alzheimer’s Detection
- POC Diagnostic Platform Combines Immunoassay and Molecular Testing
- Single Blood Test Could Detect Different Types of Cancer at Early Stages
- First Point-of-Care Heparin Monitoring Test Provides Results in Under 15 Minutes
- New Scoring System Predicts Risk of Developing Cancer from Common Blood Disorder
- Non-Invasive Prenatal Test for Fetal RhD Status Demonstrates 100% Accuracy
- WBC Count Could Predict Severity of COVID-19 Symptoms
- New Platelet Counting Technology to Help Labs Prevent Diagnosis Errors
- Machine Learning-Enabled Blood Test Predicts Immunotherapy Response in Lymphoma Patients
- Post-Treatment Blood Test Could Inform Future Cancer Therapy Decisions
- Cerebrospinal Fluid Test Predicts Dangerous Side Effect of Cancer Treatment
- New Test Measures Preterm Infant Immunity Using Only Two Drops of Blood
- Simple Blood Test Could Help Choose Better Treatments for Patients with Recurrent Endometrial Cancer
- Molecular Stool Test Shows Potential for Diagnosing TB in Adults with HIV
- New Test Diagnoses Bacterial Meningitis Quickly and Accurately
- Handheld Device Delivers Low-Cost TB Results in Less Than One Hour
- New AI-Based Method Improves Diagnosis of Drug-Resistant Infections
- Breakthrough Diagnostic Technology Identifies Bacterial Infections with Almost 100% Accuracy within Three Hours
- Light Signature Algorithm to Enable Faster and More Precise Medical Diagnoses
- Disposable Microchip Technology Could Selectively Detect HIV in Whole Blood Samples
- Pain-On-A-Chip Microfluidic Device Determines Types of Chronic Pain from Blood Samples
- Innovative, Label-Free Ratiometric Fluorosensor Enables More Sensitive Viral RNA Detection
- Smartphones Could Diagnose Diseases Using Infrared Scans
- Qiagen Acquires NGS Analysis Software Company Genoox
- Cepheid and Oxford Nanopore Technologies Partner on Advancing Automated Sequencing-Based Solutions
- Grifols and Tecan’s IBL Collaborate on Advanced Biomarker Panels
- New Collaboration to Advance Microbial Identification for Infectious Disease Diagnostics
- Tecan Acquires ELISA Immunoassay Assets from Revvity's Cisbio Bioassays
- Gene Panel Predicts Disease Progession for Patients with B-cell Lymphoma
- New Method Simplifies Preparation of Tumor Genomic DNA Libraries
- New Tool Developed for Diagnosis of Chronic HBV Infection
- Panel of Genetic Loci Accurately Predicts Risk of Developing Gout
- Disrupted TGFB Signaling Linked to Increased Cancer-Related Bacteria
- AI Microscope Spots Deadly Blood Clots Before They Strike
- New Technology Improves Understanding of Complex Biological Samples
- Combining Multiple Laboratory Techniques Provides Deeper Insights into Deadly Brain Tumors
- AI-Based Diagnosis System Identifies Malaria Parasites from Blood Smear Images
- Groundbreaking Chest Pain Triage Algorithm to Transform Cardiac Care
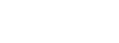
- Automated Decentralized cfDNA NGS Assay Identifies Alterations in Advanced Solid Tumors
- Mass Spectrometry Detects Bacteria Without Time-Consuming Isolation and Multiplication
- First Comprehensive Syphilis Test to Definitively Diagnose Active Infection In 10 Minutes
- Mass Spectrometry-Based Monitoring Technique to Predict and Identify Early Myeloma Relapse
- ‘Brilliantly Luminous’ Nanoscale Chemical Tool to Improve Disease Detection
- AI-Powered Platform Enables Rapid Point-of-Care Cancer Testing
- Calprotectin Testing Predicts Future Atherosclerotic Heart Disease Risk
- Low-Cost Blood Test Enables Early Alzheimer’s Detection
- POC Diagnostic Platform Combines Immunoassay and Molecular Testing
- Single Blood Test Could Detect Different Types of Cancer at Early Stages
- First Point-of-Care Heparin Monitoring Test Provides Results in Under 15 Minutes
- New Scoring System Predicts Risk of Developing Cancer from Common Blood Disorder
- Non-Invasive Prenatal Test for Fetal RhD Status Demonstrates 100% Accuracy
- WBC Count Could Predict Severity of COVID-19 Symptoms
- New Platelet Counting Technology to Help Labs Prevent Diagnosis Errors
- Machine Learning-Enabled Blood Test Predicts Immunotherapy Response in Lymphoma Patients
- Post-Treatment Blood Test Could Inform Future Cancer Therapy Decisions
- Cerebrospinal Fluid Test Predicts Dangerous Side Effect of Cancer Treatment
- New Test Measures Preterm Infant Immunity Using Only Two Drops of Blood
- Simple Blood Test Could Help Choose Better Treatments for Patients with Recurrent Endometrial Cancer
- Molecular Stool Test Shows Potential for Diagnosing TB in Adults with HIV
- New Test Diagnoses Bacterial Meningitis Quickly and Accurately
- Handheld Device Delivers Low-Cost TB Results in Less Than One Hour
- New AI-Based Method Improves Diagnosis of Drug-Resistant Infections
- Breakthrough Diagnostic Technology Identifies Bacterial Infections with Almost 100% Accuracy within Three Hours
- Light Signature Algorithm to Enable Faster and More Precise Medical Diagnoses
- Disposable Microchip Technology Could Selectively Detect HIV in Whole Blood Samples
- Pain-On-A-Chip Microfluidic Device Determines Types of Chronic Pain from Blood Samples
- Innovative, Label-Free Ratiometric Fluorosensor Enables More Sensitive Viral RNA Detection
- Smartphones Could Diagnose Diseases Using Infrared Scans
- Qiagen Acquires NGS Analysis Software Company Genoox
- Cepheid and Oxford Nanopore Technologies Partner on Advancing Automated Sequencing-Based Solutions
- Grifols and Tecan’s IBL Collaborate on Advanced Biomarker Panels
- New Collaboration to Advance Microbial Identification for Infectious Disease Diagnostics
- Tecan Acquires ELISA Immunoassay Assets from Revvity's Cisbio Bioassays
- Gene Panel Predicts Disease Progession for Patients with B-cell Lymphoma
- New Method Simplifies Preparation of Tumor Genomic DNA Libraries
- New Tool Developed for Diagnosis of Chronic HBV Infection
- Panel of Genetic Loci Accurately Predicts Risk of Developing Gout
- Disrupted TGFB Signaling Linked to Increased Cancer-Related Bacteria
- AI Microscope Spots Deadly Blood Clots Before They Strike
- New Technology Improves Understanding of Complex Biological Samples
- Combining Multiple Laboratory Techniques Provides Deeper Insights into Deadly Brain Tumors
- AI-Based Diagnosis System Identifies Malaria Parasites from Blood Smear Images
- Groundbreaking Chest Pain Triage Algorithm to Transform Cardiac Care