Expo
ADLM
view channel
view channel
view channel
view channel
view channel
view channel
view channel
view channel
view channel
Clinical Chem.Molecular DiagnosticsHematologyImmunologyMicrobiology
TechnologyIndustry
Events
Webinars
- New Reference Measurement Procedure Standardizes Nucleic Acid Amplification Test Results
- Pen-Like Tool Quickly and Non-Invasively Detects Opioids from Skin
- Simple Urine Test Could Detect Multiple Cancers at Early Stage
- Earwax Test Accurately Detects Parkinson’s by Identifying Odor Molecules
- First-Of-Its-Kind Quantitative Method Assesses Opioid Exposure in Newborns
- Molecular Diagnostics System Provides Lab-Quality Results at POC
- Cellular Signature Identifies Patients with Treatment Resistant Prostate Tumors
- MCED Could Be Valuable Supplement to Traditional Cancer Screening Approaches
- Newly-Cleared Technology a Game Changer for Diagnosis of Lyme Disease
- Innovative Liquid Biopsy Test Uses RNA to Detect Early-Stage Cancer
- First Point-of-Care Heparin Monitoring Test Provides Results in Under 15 Minutes
- New Scoring System Predicts Risk of Developing Cancer from Common Blood Disorder
- Non-Invasive Prenatal Test for Fetal RhD Status Demonstrates 100% Accuracy
- WBC Count Could Predict Severity of COVID-19 Symptoms
- New Platelet Counting Technology to Help Labs Prevent Diagnosis Errors
- Groundbreaking Lateral Flow Test Quantifies Nucleosomes in Whole Venous Blood in Minutes
- World’s First Clinical Test Predicts Best Rheumatoid Arthritis Treatment
- Blood Test Detects Organ Rejection in Heart Transplant Patients
- Liquid Biopsy Approach to Transform Diagnosis, Monitoring and Treatment of Lung Cancer
- Computational Tool Exposes Hidden Cancer DNA Changes Influencing Treatment Resistance
- Gut Microbiota Analysis Enables Early and Non-Invasive Detection of Gestational Diabetes
- Credit Card-Sized Test Boosts TB Detection in HIV Hotspots
- Fecal Metabolite Profiling Predicts Mortality in Critically Ill Patients
- Portable Molecular POC System Rules Out UTIs in Just 35 Minutes
- POC Lateral Flow Test Detects Deadly Fungal Infection Faster Than Existing Techniques
- Ultra-Sensitive Biosensor Based on Light and AI Enables Early Cancer Diagnosis
- Low-Cost Biosensing Technology Detects Disease Biomarkers in Minutes
- AI Tool Could Help Identify Specific Gut Bacterial Targets for Treatment of Diseases
- Inexpensive DNA Coated Electrode Paves Way for Disposable Diagnostics
- New Miniature Device to Transform Testing of Blood Cancer Treatments
- Lunit and Microsoft Collaborate to Advance AI-Driven Cancer Diagnosis
- AMP Releases Best Practice Recommendations to Guide Clinical Laboratories Offering HRD Testing
- Illumina Acquires SomaLogic to Accelerate Proteomics Business
- Qiagen and Gencurix Partner on Development of QIAcuity Digital PCR IVD Assay
- QIAGEN and Incyte Enter into Precision Medicine Collaboration
- Gene Panel Predicts Disease Progession for Patients with B-cell Lymphoma
- New Method Simplifies Preparation of Tumor Genomic DNA Libraries
- New Tool Developed for Diagnosis of Chronic HBV Infection
- Panel of Genetic Loci Accurately Predicts Risk of Developing Gout
- Disrupted TGFB Signaling Linked to Increased Cancer-Related Bacteria
- AI Tool Enhances Interpretation of Tissue Samples by Pathologists
- AI-Assisted Technique Tracks Cells Damaged from Injury, Aging and Disease
- Novel Fluorescent Probe Shows Potential in Precision Cancer Diagnostics and Fluorescence-Guided Surgery
- New Lab Model to Help Find Treatments for Aggressive Blood Cancer
- AI-Supported Microscopy Improves Detection of Intestinal Parasite Infections
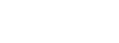
- New Reference Measurement Procedure Standardizes Nucleic Acid Amplification Test Results
- Pen-Like Tool Quickly and Non-Invasively Detects Opioids from Skin
- Simple Urine Test Could Detect Multiple Cancers at Early Stage
- Earwax Test Accurately Detects Parkinson’s by Identifying Odor Molecules
- First-Of-Its-Kind Quantitative Method Assesses Opioid Exposure in Newborns
- Molecular Diagnostics System Provides Lab-Quality Results at POC
- Cellular Signature Identifies Patients with Treatment Resistant Prostate Tumors
- MCED Could Be Valuable Supplement to Traditional Cancer Screening Approaches
- Newly-Cleared Technology a Game Changer for Diagnosis of Lyme Disease
- Innovative Liquid Biopsy Test Uses RNA to Detect Early-Stage Cancer
- First Point-of-Care Heparin Monitoring Test Provides Results in Under 15 Minutes
- New Scoring System Predicts Risk of Developing Cancer from Common Blood Disorder
- Non-Invasive Prenatal Test for Fetal RhD Status Demonstrates 100% Accuracy
- WBC Count Could Predict Severity of COVID-19 Symptoms
- New Platelet Counting Technology to Help Labs Prevent Diagnosis Errors
- Groundbreaking Lateral Flow Test Quantifies Nucleosomes in Whole Venous Blood in Minutes
- World’s First Clinical Test Predicts Best Rheumatoid Arthritis Treatment
- Blood Test Detects Organ Rejection in Heart Transplant Patients
- Liquid Biopsy Approach to Transform Diagnosis, Monitoring and Treatment of Lung Cancer
- Computational Tool Exposes Hidden Cancer DNA Changes Influencing Treatment Resistance
- Gut Microbiota Analysis Enables Early and Non-Invasive Detection of Gestational Diabetes
- Credit Card-Sized Test Boosts TB Detection in HIV Hotspots
- Fecal Metabolite Profiling Predicts Mortality in Critically Ill Patients
- Portable Molecular POC System Rules Out UTIs in Just 35 Minutes
- POC Lateral Flow Test Detects Deadly Fungal Infection Faster Than Existing Techniques
- Ultra-Sensitive Biosensor Based on Light and AI Enables Early Cancer Diagnosis
- Low-Cost Biosensing Technology Detects Disease Biomarkers in Minutes
- AI Tool Could Help Identify Specific Gut Bacterial Targets for Treatment of Diseases
- Inexpensive DNA Coated Electrode Paves Way for Disposable Diagnostics
- New Miniature Device to Transform Testing of Blood Cancer Treatments
- Lunit and Microsoft Collaborate to Advance AI-Driven Cancer Diagnosis
- AMP Releases Best Practice Recommendations to Guide Clinical Laboratories Offering HRD Testing
- Illumina Acquires SomaLogic to Accelerate Proteomics Business
- Qiagen and Gencurix Partner on Development of QIAcuity Digital PCR IVD Assay
- QIAGEN and Incyte Enter into Precision Medicine Collaboration
- Innovative New Technology to Provide Plastic-Exterior Components with Glass Interior, Presented at AACC 2015
- Portable Molecular Diagnostics System Unveiled At 2015 AACC
- Expanded Steroid Control Launched at the 2015 AACC Annual Meeting
- Eco-Friendly Immunoassay Reagents Featured at AACC 2015
- Low Cost Point-of-Care DNA Amplification Test for Chlamydia Infection Demonstrated at the 2015 AACC Annual Meeting
- Derived Exosomal Protein Biomarkers in Alzheimer’s Disease Diagnosis
- New Biochip Array Developed for ApoE4 Classification
- Cell-Free DNA Identifies Liver Transplant Patients with Acute Rejection
- New Method Tested for Early Diagnosis Pediatric Diabetic Nephropathy
- FDA-Cleared Automated Cell Counter for CSF Launched at AACC 2016
- Mesa Biotech Showcases PCR Testing Platform at Clinical Expo
- Abbott Diagnostics Displays Alinity Family of Systems
- Roche Diagnostics Highlights Latest Clinical Lab Solutions at AACC
- Sysmex Highlights Innovations in Flow Cytometry, Urinalysis and Hematology
- Ortho Clinical Diagnostics Unveils Next-Gen Analyzer at Lab Show
- Gene Panel Predicts Disease Progession for Patients with B-cell Lymphoma
- New Method Simplifies Preparation of Tumor Genomic DNA Libraries
- New Tool Developed for Diagnosis of Chronic HBV Infection
- Panel of Genetic Loci Accurately Predicts Risk of Developing Gout
- Disrupted TGFB Signaling Linked to Increased Cancer-Related Bacteria
- All Virtual 2020 AACC Uses Artificial Intelligence to Connect Exhibitors and Attendees
- Greiner Bio-One Showcases VACUETTE Virus Stabilization Tube for Transport and Storage of COVID-19 Swab Samples
- Stago Diagnostics Transforms Hemostasis with Innovations at AACC 2020 Virtual Event
- Roche Announces Newest Additions to Cobas Family of Analyzers at AACC 2020 Virtual Event
- Seegene Highlights High-Throughput, Single Tube 8-Plex RT-PCR Test for Flu A, Flu B, RSV and COVID-19 with Dual Internal Controls
- New Test that Measures Antibodies in Girls Who Have Received HPV Vaccine Presented at AACC 2021
- Novel Insights on COVID-19 Vaccines and Virus Evolution, AI in the Clinic, and Miniaturization of Diagnostic Platforms Explored at AACC 2021
- Seegene Unveils New STARlet-AIOS All-in-One Solution for All Molecular Testing at AACC 2021
- Group K Diagnostics Demonstrates KromaHealth Kit that Enables Lab-Quality Results at Point-of-Care
- Fluxergy Introduces First-of-Its-Kind Multi-Modal Laboratory Platform That Diagnoses COVID-19 On-Site in 60 Minutes
- Faster Method Diagnoses Pediatric Urinary Tract Infections
- Tianlong Showcases Integrated PCR Lab Solutions at AACC 2022
- Cellavision Introduces New Workflow Solution for Low-Volume Hematology Labs at AACC 2022
- Advanced Instruments Introduces New Automated Osmometer at AACC 2022
- Innovative Smartphone and AI-Based Tests Featured at AACC 2022
- Ground-Breaking Phage-Based Diagnostic Kit for Laboratory Tuberculosis Testing Presented at AACC 2023
- Laboratory Experts Show How They Are Leading the Way on Global Trends
- Unique Competition Focuses on Using Data Science to Forecast Preanalytical Errors
- Best Approach to Infectious Disease Serology Testing for Laboratorians and Clinicians Discussed at AACC 2023
- Breaking Research Throws Light on COVID, Flu, and RSV Co-Infections
- Cytovale Demonstrates 8-Minute Sepsis Diagnostic Test
- EKF Diagnostics Showcases Range of Diabetes and Hematology POC Devices
- Inpeco Launches Next-Generation Total Lab Automation System
- Tianlong Showcases Latest Products in Molecular Diagnostics, Biochemistry, Immunology and POC Testing
- Messe Düsseldorf Promotes International “MEDICAlliance” Portfolio at ADLM 2024
- AI Tool Enhances Interpretation of Tissue Samples by Pathologists
- AI-Assisted Technique Tracks Cells Damaged from Injury, Aging and Disease
- Novel Fluorescent Probe Shows Potential in Precision Cancer Diagnostics and Fluorescence-Guided Surgery
- New Lab Model to Help Find Treatments for Aggressive Blood Cancer
- AI-Supported Microscopy Improves Detection of Intestinal Parasite Infections