Expo
view channel
view channel
view channel
view channel
view channel
view channel
view channel
view channel
view channel
Clinical Chem.Molecular DiagnosticsHematology
MicrobiologyPathologyTechnologyIndustry
Events
- Integrated Chemistry and Immunoassay Analyzer with Extensive Assay Menu Offers Flexibility, Scalability and Data Commutability
- Rapid Drug Test to Improve Treatment for Patients Presenting to Hospital
- AI Model Detects Cancer at Lightning Speed through Sugar Analyses
- First-Ever Blood-Powered Chip Offers Real-Time Health Monitoring
- New ADLM Guidance Provides Expert Recommendations on Clinical Testing For Respiratory Viral Infections
- Handheld Device Puts Power of Lab-Based Diagnostic Testing in the Palm of Your Hand
- Protein Signatures in Blood Can Predict Risk of Developing More Than 60 Diseases
- Portable PCR Platform to Detect Multiple Pathogenic Bacteria Targets and Antibiotic Susceptibility at POC
- World’s First Rapid Diagnostic Test Detects Stroke within Minutes at POC
- Exhaled-Breath Test Shows Promise for Detection of Lung Cancer
- New Hematology Analyzers Deliver Combined ESR and CBC/DIFF Results in 60 Seconds
- Next Generation Instrument Screens for Hemoglobin Disorders in Newborns
- First 4-in-1 Nucleic Acid Test for Arbovirus Screening to Reduce Risk of Transfusion-Transmitted Infections
- POC Finger-Prick Blood Test Determines Risk of Neutropenic Sepsis in Patients Undergoing Chemotherapy
- First Affordable and Rapid Test for Beta Thalassemia Demonstrates 99% Diagnostic Accuracy
- Portable Device Analyzes White Blood Cell Activity to Monitor Cancer Patients’ Health
- New Test Detects Return of Blood Cancer a Year Earlier
- Universal Blood Test Could Predict Organ Transplant Outcomes with Unprecedented Accuracy
- AI Tool Predicts Cancer Patients’ Response to Immunotherapy
- Molecular Profiling Improves Diagnosis for Children with High Risk Cancers
- New Rapid Method for Determining Virus Infectivity Could Revolutionize Response to Future Pandemics
- Novel Molecular Test to Help Prevent and Control Multi Drug-Resistant Fungal Pathogen in Healthcare Settings
- Innovative C. Difficile Diagnostic Test Provides Both GDH and Toxin Results within 30 Minutes
- Rapid UTI Test Cuts Detection Time from 3 days to 45 Minutes
- POC STI Test Shortens Time from ED Arrival to Test Results
- New Diagnostic System Achieves PCR Testing Accuracy
- DNA Biosensor Enables Early Diagnosis of Cervical Cancer
- Self-Heating Microfluidic Devices Can Detect Diseases in Tiny Blood or Fluid Samples
- Breakthrough in Diagnostic Technology Could Make On-The-Spot Testing Widely Accessible
- First of Its Kind Technology Detects Glucose in Human Saliva
- Global Urinalysis Market Driven by Technological Advancements in POC Testing
- Roche and Hitachi High-Tech Extend 46-Year Partnership for Breakthroughs in Diagnostic Testing
- Danaher and Johns Hopkins University Collaborate to Improve Neurological Diagnosis
- Beckman Coulter and MeMed Expand Host Immune Response Diagnostics Partnership
- Thermo Fisher and Bio-Techne Enter Into Strategic Distribution Agreement for Europe
- Gene Panel Predicts Disease Progession for Patients with B-cell Lymphoma
- New Method Simplifies Preparation of Tumor Genomic DNA Libraries
- New Tool Developed for Diagnosis of Chronic HBV Infection
- Panel of Genetic Loci Accurately Predicts Risk of Developing Gout
- Disrupted TGFB Signaling Linked to Increased Cancer-Related Bacteria
- Computational Tool Integrates Transcriptomic Data for Improved Breast Cancer Diagnosis and Treatment
- AI Identifies Drug-Resistant Typhoid-Like Infection from Microscopy Images within Hours
- Whole-Slide Imaging System Enables Pathologists to Diagnose Patients Using Digital Images
- New Highly-Sensitive Test to Help More Easily Diagnose B-Cell Lymphoma
- Liver Biopsy Reveals Early Signs of Rapid Metastasis in Pancreatic Cancer Patients
Expo
view channel
view channel
view channel
view channel
view channel
view channel
view channel
view channel
view channel
Clinical Chem.Molecular DiagnosticsHematology
MicrobiologyPathologyTechnologyIndustry
Events
Advertise with Us
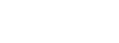
- Integrated Chemistry and Immunoassay Analyzer with Extensive Assay Menu Offers Flexibility, Scalability and Data Commutability
- Rapid Drug Test to Improve Treatment for Patients Presenting to Hospital
- AI Model Detects Cancer at Lightning Speed through Sugar Analyses
- First-Ever Blood-Powered Chip Offers Real-Time Health Monitoring
- New ADLM Guidance Provides Expert Recommendations on Clinical Testing For Respiratory Viral Infections
- Handheld Device Puts Power of Lab-Based Diagnostic Testing in the Palm of Your Hand
- Protein Signatures in Blood Can Predict Risk of Developing More Than 60 Diseases
- Portable PCR Platform to Detect Multiple Pathogenic Bacteria Targets and Antibiotic Susceptibility at POC
- World’s First Rapid Diagnostic Test Detects Stroke within Minutes at POC
- Exhaled-Breath Test Shows Promise for Detection of Lung Cancer
- New Hematology Analyzers Deliver Combined ESR and CBC/DIFF Results in 60 Seconds
- Next Generation Instrument Screens for Hemoglobin Disorders in Newborns
- First 4-in-1 Nucleic Acid Test for Arbovirus Screening to Reduce Risk of Transfusion-Transmitted Infections
- POC Finger-Prick Blood Test Determines Risk of Neutropenic Sepsis in Patients Undergoing Chemotherapy
- First Affordable and Rapid Test for Beta Thalassemia Demonstrates 99% Diagnostic Accuracy
- Portable Device Analyzes White Blood Cell Activity to Monitor Cancer Patients’ Health
- New Test Detects Return of Blood Cancer a Year Earlier
- Universal Blood Test Could Predict Organ Transplant Outcomes with Unprecedented Accuracy
- AI Tool Predicts Cancer Patients’ Response to Immunotherapy
- Molecular Profiling Improves Diagnosis for Children with High Risk Cancers
- New Rapid Method for Determining Virus Infectivity Could Revolutionize Response to Future Pandemics
- Novel Molecular Test to Help Prevent and Control Multi Drug-Resistant Fungal Pathogen in Healthcare Settings
- Innovative C. Difficile Diagnostic Test Provides Both GDH and Toxin Results within 30 Minutes
- Rapid UTI Test Cuts Detection Time from 3 days to 45 Minutes
- POC STI Test Shortens Time from ED Arrival to Test Results
- New Diagnostic System Achieves PCR Testing Accuracy
- DNA Biosensor Enables Early Diagnosis of Cervical Cancer
- Self-Heating Microfluidic Devices Can Detect Diseases in Tiny Blood or Fluid Samples
- Breakthrough in Diagnostic Technology Could Make On-The-Spot Testing Widely Accessible
- First of Its Kind Technology Detects Glucose in Human Saliva
- Global Urinalysis Market Driven by Technological Advancements in POC Testing
- Roche and Hitachi High-Tech Extend 46-Year Partnership for Breakthroughs in Diagnostic Testing
- Danaher and Johns Hopkins University Collaborate to Improve Neurological Diagnosis
- Beckman Coulter and MeMed Expand Host Immune Response Diagnostics Partnership
- Thermo Fisher and Bio-Techne Enter Into Strategic Distribution Agreement for Europe
- Gene Panel Predicts Disease Progession for Patients with B-cell Lymphoma
- New Method Simplifies Preparation of Tumor Genomic DNA Libraries
- New Tool Developed for Diagnosis of Chronic HBV Infection
- Panel of Genetic Loci Accurately Predicts Risk of Developing Gout
- Disrupted TGFB Signaling Linked to Increased Cancer-Related Bacteria
- Computational Tool Integrates Transcriptomic Data for Improved Breast Cancer Diagnosis and Treatment
- AI Identifies Drug-Resistant Typhoid-Like Infection from Microscopy Images within Hours
- Whole-Slide Imaging System Enables Pathologists to Diagnose Patients Using Digital Images
- New Highly-Sensitive Test to Help More Easily Diagnose B-Cell Lymphoma
- Liver Biopsy Reveals Early Signs of Rapid Metastasis in Pancreatic Cancer Patients