Expo
view channel
view channel
view channel
view channel
view channel
view channel
view channel
view channel
view channel
Clinical Chem.Molecular DiagnosticsHematologyImmunologyMicrobiology
TechnologyIndustry
Events
- ‘Brilliantly Luminous’ Nanoscale Chemical Tool to Improve Disease Detection
- Low-Cost Portable Screening Test to Transform Kidney Disease Detection
- New Method Uses Pulsed Infrared Light to Find Cancer's 'Fingerprints' In Blood Plasma
- Carbon Nanotubes Help Build Highly Accurate Sensors for Continuous Health Monitoring
- Paper-Based Device Boosts HIV Test Accuracy from Dried Blood Samples
- Spinal Fluid Biomarker for Parkinson’s Disease Offers Early and Accurate Diagnosis
- Revolutionary Blood Test Detects 30 Different Types of Cancers with 98% Accuracy
- Simple Blood Test Better Predicts Heart Disease Risk
- New Blood Test Detects 12 Common Cancers Before Symptoms Appear
- Blood Test Could Predict Relapse of Autoimmune Blood Vessel Disease
- Non-Invasive Prenatal Test for Fetal RhD Status Demonstrates 100% Accuracy
- WBC Count Could Predict Severity of COVID-19 Symptoms
- New Platelet Counting Technology to Help Labs Prevent Diagnosis Errors
- Streamlined Approach to Testing for Heparin-Induced Thrombocytopenia Improves Diagnostic Accuracy
- POC Hemostasis System Could Help Prevent Maternal Deaths
- Machine Learning-Enabled Blood Test Predicts Immunotherapy Response in Lymphoma Patients
- Post-Treatment Blood Test Could Inform Future Cancer Therapy Decisions
- Cerebrospinal Fluid Test Predicts Dangerous Side Effect of Cancer Treatment
- New Test Measures Preterm Infant Immunity Using Only Two Drops of Blood
- Simple Blood Test Could Help Choose Better Treatments for Patients with Recurrent Endometrial Cancer
- New AI-Based Method Improves Diagnosis of Drug-Resistant Infections
- Breakthrough Diagnostic Technology Identifies Bacterial Infections with Almost 100% Accuracy within Three Hours
- Innovative ID/AST System to Help Diagnose Infectious Diseases and Combat AMR
- Gastrointestinal Panel Delivers Rapid Detection of Five Common Bacterial Pathogens for Outpatient Use
- Rapid PCR Testing in ICU Improves Antibiotic Stewardship
- Pain-On-A-Chip Microfluidic Device Determines Types of Chronic Pain from Blood Samples
- Innovative, Label-Free Ratiometric Fluorosensor Enables More Sensitive Viral RNA Detection
- Smartphones Could Diagnose Diseases Using Infrared Scans
- Novel Sensor Technology to Enable Early Diagnoses of Metabolic and Cardiovascular Disorders
- 3D Printing Breakthrough Enables Large Scale Development of Tiny Microfluidic Devices
- Grifols and Tecan’s IBL Collaborate on Advanced Biomarker Panels
- New Collaboration to Advance Microbial Identification for Infectious Disease Diagnostics
- Tecan Acquires ELISA Immunoassay Assets from Revvity's Cisbio Bioassays
- Leica Biosystems and Bio-Techne Expand Spatial Multiomic Collaboration
- Philips and Ibex Expand Partnership to Enhance AI-Enabled Pathology Workflows
- Gene Panel Predicts Disease Progession for Patients with B-cell Lymphoma
- New Method Simplifies Preparation of Tumor Genomic DNA Libraries
- New Tool Developed for Diagnosis of Chronic HBV Infection
- Panel of Genetic Loci Accurately Predicts Risk of Developing Gout
- Disrupted TGFB Signaling Linked to Increased Cancer-Related Bacteria
- World’s First AI Model for Thyroid Cancer Diagnosis Achieves Over 90% Accuracy
- Breakthrough Diagnostic Approach to Significantly Improve TB Detection
- Rapid, Ultra-Sensitive, PCR-Free Detection Method Makes Genetic Analysis More Accessible
- Spit Test More Accurate at Identifying Future Prostate Cancer Risk
- DNA Nanotechnology Boosts Sensitivity of Test Strips
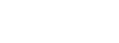
- ‘Brilliantly Luminous’ Nanoscale Chemical Tool to Improve Disease Detection
- Low-Cost Portable Screening Test to Transform Kidney Disease Detection
- New Method Uses Pulsed Infrared Light to Find Cancer's 'Fingerprints' In Blood Plasma
- Carbon Nanotubes Help Build Highly Accurate Sensors for Continuous Health Monitoring
- Paper-Based Device Boosts HIV Test Accuracy from Dried Blood Samples
- Spinal Fluid Biomarker for Parkinson’s Disease Offers Early and Accurate Diagnosis
- Revolutionary Blood Test Detects 30 Different Types of Cancers with 98% Accuracy
- Simple Blood Test Better Predicts Heart Disease Risk
- New Blood Test Detects 12 Common Cancers Before Symptoms Appear
- Blood Test Could Predict Relapse of Autoimmune Blood Vessel Disease
- Non-Invasive Prenatal Test for Fetal RhD Status Demonstrates 100% Accuracy
- WBC Count Could Predict Severity of COVID-19 Symptoms
- New Platelet Counting Technology to Help Labs Prevent Diagnosis Errors
- Streamlined Approach to Testing for Heparin-Induced Thrombocytopenia Improves Diagnostic Accuracy
- POC Hemostasis System Could Help Prevent Maternal Deaths
- Machine Learning-Enabled Blood Test Predicts Immunotherapy Response in Lymphoma Patients
- Post-Treatment Blood Test Could Inform Future Cancer Therapy Decisions
- Cerebrospinal Fluid Test Predicts Dangerous Side Effect of Cancer Treatment
- New Test Measures Preterm Infant Immunity Using Only Two Drops of Blood
- Simple Blood Test Could Help Choose Better Treatments for Patients with Recurrent Endometrial Cancer
- New AI-Based Method Improves Diagnosis of Drug-Resistant Infections
- Breakthrough Diagnostic Technology Identifies Bacterial Infections with Almost 100% Accuracy within Three Hours
- Innovative ID/AST System to Help Diagnose Infectious Diseases and Combat AMR
- Gastrointestinal Panel Delivers Rapid Detection of Five Common Bacterial Pathogens for Outpatient Use
- Rapid PCR Testing in ICU Improves Antibiotic Stewardship
- Pain-On-A-Chip Microfluidic Device Determines Types of Chronic Pain from Blood Samples
- Innovative, Label-Free Ratiometric Fluorosensor Enables More Sensitive Viral RNA Detection
- Smartphones Could Diagnose Diseases Using Infrared Scans
- Novel Sensor Technology to Enable Early Diagnoses of Metabolic and Cardiovascular Disorders
- 3D Printing Breakthrough Enables Large Scale Development of Tiny Microfluidic Devices
- Grifols and Tecan’s IBL Collaborate on Advanced Biomarker Panels
- New Collaboration to Advance Microbial Identification for Infectious Disease Diagnostics
- Tecan Acquires ELISA Immunoassay Assets from Revvity's Cisbio Bioassays
- Leica Biosystems and Bio-Techne Expand Spatial Multiomic Collaboration
- Philips and Ibex Expand Partnership to Enhance AI-Enabled Pathology Workflows
- Gene Panel Predicts Disease Progession for Patients with B-cell Lymphoma
- New Method Simplifies Preparation of Tumor Genomic DNA Libraries
- New Tool Developed for Diagnosis of Chronic HBV Infection
- Panel of Genetic Loci Accurately Predicts Risk of Developing Gout
- Disrupted TGFB Signaling Linked to Increased Cancer-Related Bacteria
- World’s First AI Model for Thyroid Cancer Diagnosis Achieves Over 90% Accuracy
- Breakthrough Diagnostic Approach to Significantly Improve TB Detection
- Rapid, Ultra-Sensitive, PCR-Free Detection Method Makes Genetic Analysis More Accessible
- Spit Test More Accurate at Identifying Future Prostate Cancer Risk
- DNA Nanotechnology Boosts Sensitivity of Test Strips