Expo
view channel
view channel
view channel
view channel
view channel
view channel
view channel
view channel
view channel
Clinical Chem.Molecular DiagnosticsHematologyImmunology
PathologyTechnologyIndustry
Events
- First Comprehensive Syphilis Test to Definitively Diagnose Active Infection In 10 Minutes
- Mass Spectrometry-Based Monitoring Technique to Predict and Identify Early Myeloma Relapse
- ‘Brilliantly Luminous’ Nanoscale Chemical Tool to Improve Disease Detection
- Low-Cost Portable Screening Test to Transform Kidney Disease Detection
- New Method Uses Pulsed Infrared Light to Find Cancer's 'Fingerprints' In Blood Plasma
- New Molecular Label to Help Develop Simpler and Faster Tuberculosis Tests
- Biomarker Discovery Paves Way for Blood Tests to Detect and Treat Osteoarthritis
- Liquid Biopsy Assay Detects Recurrence in CRC Patients Prior to Imaging
- Ultra Fast Synovial Fluid Test Diagnoses Osteoarthritis and Rheumatoid Arthritis In 10 Minutes
- Genetic-Based Tool Predicts Survival Outcomes of Pancreatic Cancer Patients
- New Scoring System Predicts Risk of Developing Cancer from Common Blood Disorder
- Non-Invasive Prenatal Test for Fetal RhD Status Demonstrates 100% Accuracy
- WBC Count Could Predict Severity of COVID-19 Symptoms
- New Platelet Counting Technology to Help Labs Prevent Diagnosis Errors
- Streamlined Approach to Testing for Heparin-Induced Thrombocytopenia Improves Diagnostic Accuracy
- Machine Learning-Enabled Blood Test Predicts Immunotherapy Response in Lymphoma Patients
- Post-Treatment Blood Test Could Inform Future Cancer Therapy Decisions
- Cerebrospinal Fluid Test Predicts Dangerous Side Effect of Cancer Treatment
- New Test Measures Preterm Infant Immunity Using Only Two Drops of Blood
- Simple Blood Test Could Help Choose Better Treatments for Patients with Recurrent Endometrial Cancer
- New Test Diagnoses Bacterial Meningitis Quickly and Accurately
- Handheld Device Delivers Low-Cost TB Results in Less Than One Hour
- New AI-Based Method Improves Diagnosis of Drug-Resistant Infections
- Breakthrough Diagnostic Technology Identifies Bacterial Infections with Almost 100% Accuracy within Three Hours
- Innovative ID/AST System to Help Diagnose Infectious Diseases and Combat AMR
- Light Signature Algorithm to Enable Faster and More Precise Medical Diagnoses
- Disposable Microchip Technology Could Selectively Detect HIV in Whole Blood Samples
- Pain-On-A-Chip Microfluidic Device Determines Types of Chronic Pain from Blood Samples
- Innovative, Label-Free Ratiometric Fluorosensor Enables More Sensitive Viral RNA Detection
- Smartphones Could Diagnose Diseases Using Infrared Scans
- Grifols and Tecan’s IBL Collaborate on Advanced Biomarker Panels
- New Collaboration to Advance Microbial Identification for Infectious Disease Diagnostics
- Tecan Acquires ELISA Immunoassay Assets from Revvity's Cisbio Bioassays
- Leica Biosystems and Bio-Techne Expand Spatial Multiomic Collaboration
- Philips and Ibex Expand Partnership to Enhance AI-Enabled Pathology Workflows
- Gene Panel Predicts Disease Progession for Patients with B-cell Lymphoma
- New Method Simplifies Preparation of Tumor Genomic DNA Libraries
- New Tool Developed for Diagnosis of Chronic HBV Infection
- Panel of Genetic Loci Accurately Predicts Risk of Developing Gout
- Disrupted TGFB Signaling Linked to Increased Cancer-Related Bacteria
- AI-Based Liquid Biopsy Approach to Revolutionize Brain Cancer Detection
- AI-Driven Analysis of Digital Pathology Images to Improve Pediatric Sarcoma Subtyping
- AI-Based Model Predicts Kidney Cancer Therapy Response
- Sensitive and Specific DUB Enzyme Assay Kits Require Minimal Setup Without Substrate Preparation
- World’s First AI Model for Thyroid Cancer Diagnosis Achieves Over 90% Accuracy
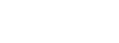
- First Comprehensive Syphilis Test to Definitively Diagnose Active Infection In 10 Minutes
- Mass Spectrometry-Based Monitoring Technique to Predict and Identify Early Myeloma Relapse
- ‘Brilliantly Luminous’ Nanoscale Chemical Tool to Improve Disease Detection
- Low-Cost Portable Screening Test to Transform Kidney Disease Detection
- New Method Uses Pulsed Infrared Light to Find Cancer's 'Fingerprints' In Blood Plasma
- New Molecular Label to Help Develop Simpler and Faster Tuberculosis Tests
- Biomarker Discovery Paves Way for Blood Tests to Detect and Treat Osteoarthritis
- Liquid Biopsy Assay Detects Recurrence in CRC Patients Prior to Imaging
- Ultra Fast Synovial Fluid Test Diagnoses Osteoarthritis and Rheumatoid Arthritis In 10 Minutes
- Genetic-Based Tool Predicts Survival Outcomes of Pancreatic Cancer Patients
- New Scoring System Predicts Risk of Developing Cancer from Common Blood Disorder
- Non-Invasive Prenatal Test for Fetal RhD Status Demonstrates 100% Accuracy
- WBC Count Could Predict Severity of COVID-19 Symptoms
- New Platelet Counting Technology to Help Labs Prevent Diagnosis Errors
- Streamlined Approach to Testing for Heparin-Induced Thrombocytopenia Improves Diagnostic Accuracy
- Machine Learning-Enabled Blood Test Predicts Immunotherapy Response in Lymphoma Patients
- Post-Treatment Blood Test Could Inform Future Cancer Therapy Decisions
- Cerebrospinal Fluid Test Predicts Dangerous Side Effect of Cancer Treatment
- New Test Measures Preterm Infant Immunity Using Only Two Drops of Blood
- Simple Blood Test Could Help Choose Better Treatments for Patients with Recurrent Endometrial Cancer
- New Test Diagnoses Bacterial Meningitis Quickly and Accurately
- Handheld Device Delivers Low-Cost TB Results in Less Than One Hour
- New AI-Based Method Improves Diagnosis of Drug-Resistant Infections
- Breakthrough Diagnostic Technology Identifies Bacterial Infections with Almost 100% Accuracy within Three Hours
- Innovative ID/AST System to Help Diagnose Infectious Diseases and Combat AMR
- Light Signature Algorithm to Enable Faster and More Precise Medical Diagnoses
- Disposable Microchip Technology Could Selectively Detect HIV in Whole Blood Samples
- Pain-On-A-Chip Microfluidic Device Determines Types of Chronic Pain from Blood Samples
- Innovative, Label-Free Ratiometric Fluorosensor Enables More Sensitive Viral RNA Detection
- Smartphones Could Diagnose Diseases Using Infrared Scans
- Grifols and Tecan’s IBL Collaborate on Advanced Biomarker Panels
- New Collaboration to Advance Microbial Identification for Infectious Disease Diagnostics
- Tecan Acquires ELISA Immunoassay Assets from Revvity's Cisbio Bioassays
- Leica Biosystems and Bio-Techne Expand Spatial Multiomic Collaboration
- Philips and Ibex Expand Partnership to Enhance AI-Enabled Pathology Workflows
- Gene Panel Predicts Disease Progession for Patients with B-cell Lymphoma
- New Method Simplifies Preparation of Tumor Genomic DNA Libraries
- New Tool Developed for Diagnosis of Chronic HBV Infection
- Panel of Genetic Loci Accurately Predicts Risk of Developing Gout
- Disrupted TGFB Signaling Linked to Increased Cancer-Related Bacteria
- AI-Based Liquid Biopsy Approach to Revolutionize Brain Cancer Detection
- AI-Driven Analysis of Digital Pathology Images to Improve Pediatric Sarcoma Subtyping
- AI-Based Model Predicts Kidney Cancer Therapy Response
- Sensitive and Specific DUB Enzyme Assay Kits Require Minimal Setup Without Substrate Preparation
- World’s First AI Model for Thyroid Cancer Diagnosis Achieves Over 90% Accuracy