Expo
view channel
view channel
view channel
view channel
view channel
view channel
view channel
view channel
view channel
Clinical Chem.Molecular DiagnosticsHematologyImmunology
PathologyTechnologyIndustry
Events
Webinars
- New Reference Measurement Procedure Standardizes Nucleic Acid Amplification Test Results
- Pen-Like Tool Quickly and Non-Invasively Detects Opioids from Skin
- Simple Urine Test Could Detect Multiple Cancers at Early Stage
- Earwax Test Accurately Detects Parkinson’s by Identifying Odor Molecules
- First-Of-Its-Kind Quantitative Method Assesses Opioid Exposure in Newborns
- New Technique for Measuring Acidic Glycan in Blood Simplifies Schizophrenia Diagnosis
- Injury Molecular Fingerprint Enables Real-Time Diagnostics for On-Site Treatment
- Blood Test Could Predict Likelihood of Breast Cancer Spreading to The Bone
- New Infectious Disease Analytics Platform Speeds Up Clinical Decision-Making at POC
- Genetic Test Could Predict Poor Outcomes in Lung Transplant Patients
- First Point-of-Care Heparin Monitoring Test Provides Results in Under 15 Minutes
- New Scoring System Predicts Risk of Developing Cancer from Common Blood Disorder
- Non-Invasive Prenatal Test for Fetal RhD Status Demonstrates 100% Accuracy
- WBC Count Could Predict Severity of COVID-19 Symptoms
- New Platelet Counting Technology to Help Labs Prevent Diagnosis Errors
- Liquid Biopsy Approach to Transform Diagnosis, Monitoring and Treatment of Lung Cancer
- Computational Tool Exposes Hidden Cancer DNA Changes Influencing Treatment Resistance
- New Tool Detects Breast Cancer Relapses Five Years in Advance
- T Cells in Blood Can Detect Parkinson's Years Before Diagnosis
- POC Diagnostic Platform Performs Immune Analysis Using One Drop of Fingertip Blood
- Gut Microbiota Analysis Enables Early and Non-Invasive Detection of Gestational Diabetes
- Credit Card-Sized Test Boosts TB Detection in HIV Hotspots
- Fecal Metabolite Profiling Predicts Mortality in Critically Ill Patients
- Portable Molecular POC System Rules Out UTIs in Just 35 Minutes
- POC Lateral Flow Test Detects Deadly Fungal Infection Faster Than Existing Techniques
- New Miniature Device to Transform Testing of Blood Cancer Treatments
- Biosensing Advancement to Enable Early Detection of Disease Biomarkers at POC
- New POC Biosensing Technology Improves Detection of Molecular Biomarkers
- Enhanced Lab Data Management and AI Critical to Labs of the Future, Finds Survey
- AI-Assisted Non-DNA Based Test Identifies Viral Infections in Minutes
- AMP Releases Best Practice Recommendations to Guide Clinical Laboratories Offering HRD Testing
- Illumina Acquires SomaLogic to Accelerate Proteomics Business
- Qiagen and Gencurix Partner on Development of QIAcuity Digital PCR IVD Assay
- QIAGEN and Incyte Enter into Precision Medicine Collaboration
- bioMérieux Acquires Day Zero Diagnostics Solutions and Technologies
- Gene Panel Predicts Disease Progession for Patients with B-cell Lymphoma
- New Method Simplifies Preparation of Tumor Genomic DNA Libraries
- New Tool Developed for Diagnosis of Chronic HBV Infection
- Panel of Genetic Loci Accurately Predicts Risk of Developing Gout
- Disrupted TGFB Signaling Linked to Increased Cancer-Related Bacteria
- AI-Driven Preliminary Testing for Pancreatic Cancer Enhances Prognosis
- Cancer Chip Accurately Predicts Patient-Specific Chemotherapy Response
- Clinical AI Solution for Automatic Breast Cancer Grading Improves Diagnostic Accuracy
- Saliva-Based Testing to Enable Early Detection of Cancer, Heart Disease or Parkinson’s
- Advances in Monkeypox Virus Diagnostics to Improve Management of Future Outbreaks
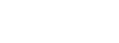
- New Reference Measurement Procedure Standardizes Nucleic Acid Amplification Test Results
- Pen-Like Tool Quickly and Non-Invasively Detects Opioids from Skin
- Simple Urine Test Could Detect Multiple Cancers at Early Stage
- Earwax Test Accurately Detects Parkinson’s by Identifying Odor Molecules
- First-Of-Its-Kind Quantitative Method Assesses Opioid Exposure in Newborns
- New Technique for Measuring Acidic Glycan in Blood Simplifies Schizophrenia Diagnosis
- Injury Molecular Fingerprint Enables Real-Time Diagnostics for On-Site Treatment
- Blood Test Could Predict Likelihood of Breast Cancer Spreading to The Bone
- New Infectious Disease Analytics Platform Speeds Up Clinical Decision-Making at POC
- Genetic Test Could Predict Poor Outcomes in Lung Transplant Patients
- First Point-of-Care Heparin Monitoring Test Provides Results in Under 15 Minutes
- New Scoring System Predicts Risk of Developing Cancer from Common Blood Disorder
- Non-Invasive Prenatal Test for Fetal RhD Status Demonstrates 100% Accuracy
- WBC Count Could Predict Severity of COVID-19 Symptoms
- New Platelet Counting Technology to Help Labs Prevent Diagnosis Errors
- Liquid Biopsy Approach to Transform Diagnosis, Monitoring and Treatment of Lung Cancer
- Computational Tool Exposes Hidden Cancer DNA Changes Influencing Treatment Resistance
- New Tool Detects Breast Cancer Relapses Five Years in Advance
- T Cells in Blood Can Detect Parkinson's Years Before Diagnosis
- POC Diagnostic Platform Performs Immune Analysis Using One Drop of Fingertip Blood
- Gut Microbiota Analysis Enables Early and Non-Invasive Detection of Gestational Diabetes
- Credit Card-Sized Test Boosts TB Detection in HIV Hotspots
- Fecal Metabolite Profiling Predicts Mortality in Critically Ill Patients
- Portable Molecular POC System Rules Out UTIs in Just 35 Minutes
- POC Lateral Flow Test Detects Deadly Fungal Infection Faster Than Existing Techniques
- New Miniature Device to Transform Testing of Blood Cancer Treatments
- Biosensing Advancement to Enable Early Detection of Disease Biomarkers at POC
- New POC Biosensing Technology Improves Detection of Molecular Biomarkers
- Enhanced Lab Data Management and AI Critical to Labs of the Future, Finds Survey
- AI-Assisted Non-DNA Based Test Identifies Viral Infections in Minutes
- AMP Releases Best Practice Recommendations to Guide Clinical Laboratories Offering HRD Testing
- Illumina Acquires SomaLogic to Accelerate Proteomics Business
- Qiagen and Gencurix Partner on Development of QIAcuity Digital PCR IVD Assay
- QIAGEN and Incyte Enter into Precision Medicine Collaboration
- bioMérieux Acquires Day Zero Diagnostics Solutions and Technologies
- Gene Panel Predicts Disease Progession for Patients with B-cell Lymphoma
- New Method Simplifies Preparation of Tumor Genomic DNA Libraries
- New Tool Developed for Diagnosis of Chronic HBV Infection
- Panel of Genetic Loci Accurately Predicts Risk of Developing Gout
- Disrupted TGFB Signaling Linked to Increased Cancer-Related Bacteria
- AI-Driven Preliminary Testing for Pancreatic Cancer Enhances Prognosis
- Cancer Chip Accurately Predicts Patient-Specific Chemotherapy Response
- Clinical AI Solution for Automatic Breast Cancer Grading Improves Diagnostic Accuracy
- Saliva-Based Testing to Enable Early Detection of Cancer, Heart Disease or Parkinson’s
- Advances in Monkeypox Virus Diagnostics to Improve Management of Future Outbreaks