Expo
view channel
view channel
view channel
view channel
view channel
view channel
view channel
view channel
view channel
Clinical Chem.Molecular DiagnosticsHematologyImmunologyMicrobiology
TechnologyIndustry
Events
- POC Biomedical Test Spins Water Droplet Using Sound Waves for Cancer Detection
- Highly Reliable Cell-Based Assay Enables Accurate Diagnosis of Endocrine Diseases
- New Blood Testing Method Detects Potent Opioids in Under Three Minutes
- Wireless Hepatitis B Test Kit Completes Screening and Data Collection in One Step
- Pain-Free, Low-Cost, Sensitive, Radiation-Free Device Detects Breast Cancer in Urine
- Simple Blood Test Could Enable First Quantitative Assessments for Future Cerebrovascular Disease
- New Genetic Testing Procedure Combined With Ultrasound Detects High Cardiovascular Risk
- Blood Samples Enhance B-Cell Lymphoma Diagnostics and Prognosis
- Blood Test Predicts Knee Osteoarthritis Eight Years Before Signs Appears On X-Rays
- Blood Test Accurately Predicts Lung Cancer Risk and Reduces Need for Scans
- First 4-in-1 Nucleic Acid Test for Arbovirus Screening to Reduce Risk of Transfusion-Transmitted Infections
- POC Finger-Prick Blood Test Determines Risk of Neutropenic Sepsis in Patients Undergoing Chemotherapy
- First Affordable and Rapid Test for Beta Thalassemia Demonstrates 99% Diagnostic Accuracy
- Handheld White Blood Cell Tracker to Enable Rapid Testing For Infections
- Smart Palm-size Optofluidic Hematology Analyzer Enables POCT of Patients’ Blood Cells
- AI Tool Precisely Matches Cancer Drugs to Patients Using Information from Each Tumor Cell
- Genetic Testing Combined With Personalized Drug Screening On Tumor Samples to Revolutionize Cancer Treatment
- Testing Method Could Help More Patients Receive Right Cancer Treatment
- Groundbreaking Test Monitors Radiation Therapy Toxicity in Cancer Patients
- State-Of-The Art Techniques to Investigate Immune Response in Deadly Strep A Infections
- Clinical Decision Support Software a Game-Changer in Antimicrobial Resistance Battle
- New CE-Marked Hepatitis Assays to Help Diagnose Infections Earlier
- 1 Hour, Direct-From-Blood Multiplex PCR Test Identifies 95% of Sepsis-Causing Pathogens
- Mouth Bacteria Test Could Predict Colon Cancer Progression
- Unique Metabolic Signature Could Enable Sepsis Diagnosis within One Hour of Blood Collection
- DNA Biosensor Enables Early Diagnosis of Cervical Cancer
- Self-Heating Microfluidic Devices Can Detect Diseases in Tiny Blood or Fluid Samples
- Breakthrough in Diagnostic Technology Could Make On-The-Spot Testing Widely Accessible
- First of Its Kind Technology Detects Glucose in Human Saliva
- Electrochemical Device Identifies People at Higher Risk for Osteoporosis Using Single Blood Drop
- ECCMID Congress Name Changes to ESCMID Global
- Bosch and Randox Partner to Make Strategic Investment in Vivalytic Analysis Platform
- Siemens to Close Fast Track Diagnostics Business
- Beckman Coulter and Fujirebio Expand Partnership on Neurodegenerative Disease Diagnostics
- Sysmex and Hitachi Collaborate on Development of New Genetic Testing Systems
- Gene Panel Predicts Disease Progession for Patients with B-cell Lymphoma
- New Method Simplifies Preparation of Tumor Genomic DNA Libraries
- New Tool Developed for Diagnosis of Chronic HBV Infection
- Panel of Genetic Loci Accurately Predicts Risk of Developing Gout
- Disrupted TGFB Signaling Linked to Increased Cancer-Related Bacteria
- Use of DICOM Images for Pathology Diagnostics Marks Significant Step towards Standardization
- First of Its Kind Universal Tool to Revolutionize Sample Collection for Diagnostic Tests
- AI-Powered Digital Imaging System to Revolutionize Cancer Diagnosis
- New Mycobacterium Tuberculosis Panel to Support Real-Time Surveillance and Combat Antimicrobial Resistance
- New Method Offers Sustainable Approach to Universal Metabolic Cancer Diagnosis
Expo
view channel
view channel
view channel
view channel
view channel
view channel
view channel
view channel
view channel
Clinical Chem.Molecular DiagnosticsHematologyImmunologyMicrobiology
TechnologyIndustry
Events
Advertise with Us
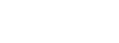
- POC Biomedical Test Spins Water Droplet Using Sound Waves for Cancer Detection
- Highly Reliable Cell-Based Assay Enables Accurate Diagnosis of Endocrine Diseases
- New Blood Testing Method Detects Potent Opioids in Under Three Minutes
- Wireless Hepatitis B Test Kit Completes Screening and Data Collection in One Step
- Pain-Free, Low-Cost, Sensitive, Radiation-Free Device Detects Breast Cancer in Urine
- Simple Blood Test Could Enable First Quantitative Assessments for Future Cerebrovascular Disease
- New Genetic Testing Procedure Combined With Ultrasound Detects High Cardiovascular Risk
- Blood Samples Enhance B-Cell Lymphoma Diagnostics and Prognosis
- Blood Test Predicts Knee Osteoarthritis Eight Years Before Signs Appears On X-Rays
- Blood Test Accurately Predicts Lung Cancer Risk and Reduces Need for Scans
- First 4-in-1 Nucleic Acid Test for Arbovirus Screening to Reduce Risk of Transfusion-Transmitted Infections
- POC Finger-Prick Blood Test Determines Risk of Neutropenic Sepsis in Patients Undergoing Chemotherapy
- First Affordable and Rapid Test for Beta Thalassemia Demonstrates 99% Diagnostic Accuracy
- Handheld White Blood Cell Tracker to Enable Rapid Testing For Infections
- Smart Palm-size Optofluidic Hematology Analyzer Enables POCT of Patients’ Blood Cells
- AI Tool Precisely Matches Cancer Drugs to Patients Using Information from Each Tumor Cell
- Genetic Testing Combined With Personalized Drug Screening On Tumor Samples to Revolutionize Cancer Treatment
- Testing Method Could Help More Patients Receive Right Cancer Treatment
- Groundbreaking Test Monitors Radiation Therapy Toxicity in Cancer Patients
- State-Of-The Art Techniques to Investigate Immune Response in Deadly Strep A Infections
- Clinical Decision Support Software a Game-Changer in Antimicrobial Resistance Battle
- New CE-Marked Hepatitis Assays to Help Diagnose Infections Earlier
- 1 Hour, Direct-From-Blood Multiplex PCR Test Identifies 95% of Sepsis-Causing Pathogens
- Mouth Bacteria Test Could Predict Colon Cancer Progression
- Unique Metabolic Signature Could Enable Sepsis Diagnosis within One Hour of Blood Collection
- DNA Biosensor Enables Early Diagnosis of Cervical Cancer
- Self-Heating Microfluidic Devices Can Detect Diseases in Tiny Blood or Fluid Samples
- Breakthrough in Diagnostic Technology Could Make On-The-Spot Testing Widely Accessible
- First of Its Kind Technology Detects Glucose in Human Saliva
- Electrochemical Device Identifies People at Higher Risk for Osteoporosis Using Single Blood Drop
- ECCMID Congress Name Changes to ESCMID Global
- Bosch and Randox Partner to Make Strategic Investment in Vivalytic Analysis Platform
- Siemens to Close Fast Track Diagnostics Business
- Beckman Coulter and Fujirebio Expand Partnership on Neurodegenerative Disease Diagnostics
- Sysmex and Hitachi Collaborate on Development of New Genetic Testing Systems
- Gene Panel Predicts Disease Progession for Patients with B-cell Lymphoma
- New Method Simplifies Preparation of Tumor Genomic DNA Libraries
- New Tool Developed for Diagnosis of Chronic HBV Infection
- Panel of Genetic Loci Accurately Predicts Risk of Developing Gout
- Disrupted TGFB Signaling Linked to Increased Cancer-Related Bacteria
- Use of DICOM Images for Pathology Diagnostics Marks Significant Step towards Standardization
- First of Its Kind Universal Tool to Revolutionize Sample Collection for Diagnostic Tests
- AI-Powered Digital Imaging System to Revolutionize Cancer Diagnosis
- New Mycobacterium Tuberculosis Panel to Support Real-Time Surveillance and Combat Antimicrobial Resistance
- New Method Offers Sustainable Approach to Universal Metabolic Cancer Diagnosis