Expo
view channel
view channel
view channel
view channel
view channel
view channel
view channel
view channel
view channel
Clinical Chem.Molecular DiagnosticsHematologyImmunologyMicrobiology
TechnologyIndustry
Events
- AI-Powered Lung Maturity Test Identifies Newborns at Higher Risk of Respiratory Distress
- AI-Powered Blood Test Accurately Detects Ovarian Cancer
- Automated Decentralized cfDNA NGS Assay Identifies Alterations in Advanced Solid Tumors
- Mass Spectrometry Detects Bacteria Without Time-Consuming Isolation and Multiplication
- First Comprehensive Syphilis Test to Definitively Diagnose Active Infection In 10 Minutes
- MCED Tests Detect Cancers in Bloodstream Three Years Prior To Diagnosis
- Revolutionary Blood Test Aims to Fast-Track Lung Cancer Patients for Targeted Therapy
- Simple Paper Test Offers Early Cancer Diagnosis
- Precision Oncology Test Predicts Patient Toxicity Before Beginning Chemotherapy
- Blood-Based Multi-Cancer Test Sets New Standard for Ultra-Sensitive and Early MRD Detection
- First Point-of-Care Heparin Monitoring Test Provides Results in Under 15 Minutes
- New Scoring System Predicts Risk of Developing Cancer from Common Blood Disorder
- Non-Invasive Prenatal Test for Fetal RhD Status Demonstrates 100% Accuracy
- WBC Count Could Predict Severity of COVID-19 Symptoms
- New Platelet Counting Technology to Help Labs Prevent Diagnosis Errors
- Treatment Switching Guided by Liquid Biopsy Blood Tests Improves Outcomes for Breast Cancer Patients
- First-Of-Its-Kind Device Profiles Newborns' Immune Function Using Single Blood Drop
- Stem Cell Test Predicts Treatment Outcome for Patients with Platinum-Resistant Ovarian Cancer
- Machine Learning-Enabled Blood Test Predicts Immunotherapy Response in Lymphoma Patients
- Post-Treatment Blood Test Could Inform Future Cancer Therapy Decisions
- Diagnostic Test Accurately Detects Colorectal Cancer by Identifying Microbial Signature in Gut Bacteria
- Rapid Bedside Test Predicts Sepsis with Over 90% Accuracy
- New Blood Test Detects Up to Five Infectious Diseases at POC
- Molecular Stool Test Shows Potential for Diagnosing TB in Adults with HIV
- New Test Diagnoses Bacterial Meningitis Quickly and Accurately
- Breath Test to Enable Early Detection of Breast Cancer
- First Ever Technology Recognizes Disease Biomarkers Directly in Menstrual Blood in Sanitary Towels
- Tiny Microlaser Sensors with Supercharged Biosensing Ability to Enable Early Disease Diagnosis
- Advanced Predictive Algorithms Identify Patients Having Undiagnosed Cancer
- Light Signature Algorithm to Enable Faster and More Precise Medical Diagnoses
- QuidelOrtho to Acquire MDx Company LEX Diagnostics
- QIAGEN Enters into New Strategic Partnerships for Expanding MRD Testing Portfolio in Oncology
- Danaher and AstraZeneca Partner on Next Generation AI-Powered Diagnostics
- EuroMedLab 2025 Showcases Latest Technologies and Innovations in Laboratory Medicine
- Qiagen Acquires NGS Analysis Software Company Genoox
- Gene Panel Predicts Disease Progession for Patients with B-cell Lymphoma
- New Method Simplifies Preparation of Tumor Genomic DNA Libraries
- New Tool Developed for Diagnosis of Chronic HBV Infection
- Panel of Genetic Loci Accurately Predicts Risk of Developing Gout
- Disrupted TGFB Signaling Linked to Increased Cancer-Related Bacteria
- New At-Home Cervical Cancer Screening Device to Increase Accessibility for Patients
- New Cancer Testing Standards to Improve Diagnostic Accuracy for Oncology Labs
- Digital Diagnostic Platform Uses Electronic Technology for Rapid Disease Detection
- New Tools Improve Imaging of Cardiac Tissue and Vessels for Earlier Disease Diagnoses
- ML Algorithm Accurately Identifies Cancer-Specific Structural in Long-Read DNA Sequencing Data
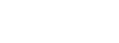
- AI-Powered Lung Maturity Test Identifies Newborns at Higher Risk of Respiratory Distress
- AI-Powered Blood Test Accurately Detects Ovarian Cancer
- Automated Decentralized cfDNA NGS Assay Identifies Alterations in Advanced Solid Tumors
- Mass Spectrometry Detects Bacteria Without Time-Consuming Isolation and Multiplication
- First Comprehensive Syphilis Test to Definitively Diagnose Active Infection In 10 Minutes
- MCED Tests Detect Cancers in Bloodstream Three Years Prior To Diagnosis
- Revolutionary Blood Test Aims to Fast-Track Lung Cancer Patients for Targeted Therapy
- Simple Paper Test Offers Early Cancer Diagnosis
- Precision Oncology Test Predicts Patient Toxicity Before Beginning Chemotherapy
- Blood-Based Multi-Cancer Test Sets New Standard for Ultra-Sensitive and Early MRD Detection
- First Point-of-Care Heparin Monitoring Test Provides Results in Under 15 Minutes
- New Scoring System Predicts Risk of Developing Cancer from Common Blood Disorder
- Non-Invasive Prenatal Test for Fetal RhD Status Demonstrates 100% Accuracy
- WBC Count Could Predict Severity of COVID-19 Symptoms
- New Platelet Counting Technology to Help Labs Prevent Diagnosis Errors
- Treatment Switching Guided by Liquid Biopsy Blood Tests Improves Outcomes for Breast Cancer Patients
- First-Of-Its-Kind Device Profiles Newborns' Immune Function Using Single Blood Drop
- Stem Cell Test Predicts Treatment Outcome for Patients with Platinum-Resistant Ovarian Cancer
- Machine Learning-Enabled Blood Test Predicts Immunotherapy Response in Lymphoma Patients
- Post-Treatment Blood Test Could Inform Future Cancer Therapy Decisions
- Diagnostic Test Accurately Detects Colorectal Cancer by Identifying Microbial Signature in Gut Bacteria
- Rapid Bedside Test Predicts Sepsis with Over 90% Accuracy
- New Blood Test Detects Up to Five Infectious Diseases at POC
- Molecular Stool Test Shows Potential for Diagnosing TB in Adults with HIV
- New Test Diagnoses Bacterial Meningitis Quickly and Accurately
- Breath Test to Enable Early Detection of Breast Cancer
- First Ever Technology Recognizes Disease Biomarkers Directly in Menstrual Blood in Sanitary Towels
- Tiny Microlaser Sensors with Supercharged Biosensing Ability to Enable Early Disease Diagnosis
- Advanced Predictive Algorithms Identify Patients Having Undiagnosed Cancer
- Light Signature Algorithm to Enable Faster and More Precise Medical Diagnoses
- QuidelOrtho to Acquire MDx Company LEX Diagnostics
- QIAGEN Enters into New Strategic Partnerships for Expanding MRD Testing Portfolio in Oncology
- Danaher and AstraZeneca Partner on Next Generation AI-Powered Diagnostics
- EuroMedLab 2025 Showcases Latest Technologies and Innovations in Laboratory Medicine
- Qiagen Acquires NGS Analysis Software Company Genoox
- Gene Panel Predicts Disease Progession for Patients with B-cell Lymphoma
- New Method Simplifies Preparation of Tumor Genomic DNA Libraries
- New Tool Developed for Diagnosis of Chronic HBV Infection
- Panel of Genetic Loci Accurately Predicts Risk of Developing Gout
- Disrupted TGFB Signaling Linked to Increased Cancer-Related Bacteria
- New At-Home Cervical Cancer Screening Device to Increase Accessibility for Patients
- New Cancer Testing Standards to Improve Diagnostic Accuracy for Oncology Labs
- Digital Diagnostic Platform Uses Electronic Technology for Rapid Disease Detection
- New Tools Improve Imaging of Cardiac Tissue and Vessels for Earlier Disease Diagnoses
- ML Algorithm Accurately Identifies Cancer-Specific Structural in Long-Read DNA Sequencing Data